eddy2017
Elite Member
- Joined
- Oct 27, 2017
- Messages
- 2,525
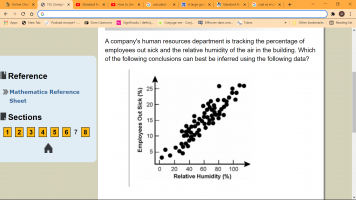
- High values of relative humidity cause people to become sick.
- When many employees are out sick, the relative humidity tends to be high.
- Many employees are getting sick from the mold and mildew in the building.
- The negative affect of overcast, sunless days is influencing employees' health
I looked up the answer before I pasted here( which I never use even if the exercise carries an answer because that defeats the whole purpose of my reason to post here. Besides, you may see the answer and not know a blessed thing about why that is the answer.
well, this is a typical case
the answer given as correct is B >When many employees are out sick, the relative humidity tends to be high.
I have studied scatter plots, but what i have learned is not enough to see why is B the answer
on the y axis of the scatter plot th highest percent of sickouts is 25 % and the relative humidity let's say it goes up from 80 % but I don't see the connection. Where both parameters meet the scatterplot dots are not so tightly together. there are tighter spots.
What am I missing in this picture?
thanks in advance,
eddy